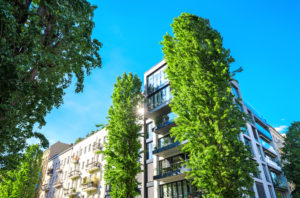
By Daniele Vergari, Gianluca Natalini, Stella Fumarola, CRIF
One of the key objectives within the EeDaPP project is to investigate the correlation between energy efficiency and credit risk, measuring how this affects obligor’s credit risk and thus a “green” mortgage portfolio performance.
To this end, CRIF, closely working with University of Venice “Ca’ Foscari” and the other EeDaPP consortium partners, will be preparing the ground for the quantitative analyses. These will focus on the two main components of credit risk and its cost trying to consolidate our previous research evidence:
In order to make the study as sound and robust as possible, different market players and stakeholders are involved firstly in the creation of the analysis data pool:
Once the pool data is available, a rigorous process of data normalization (needed to make data coming from different sources and / or institutions comparable and coherent) takes place followed then by the quantitative (econometric) analyses. The overall process is summarized in the following five steps:
Crif has already carried out a preliminary assessment of the impact on credit risk of energy class using a sample of about 18.000 mortgage loans for which all customer level (e.g. age, marital status, residential status, etc.) and contract level information (e.g. property EPC, loan amount, property value, downpayment amount, term, etc.) was available. Credit performance was also available allowing to observe credit risk differences between energy efficient and non-energy efficient obligors. In order to take in due account potential customer profile differences, the portfolio was clustered into segments homogeneous in terms of property value and loan-to-value rate.
The analyses showed that, within the identified clusters, that energy classes A and B report a much lover one-year default rate compared to the other classes (that are twice as risky and classes A and B).
The sample size is not large enough to generate robust evidence. Further analyses and larger and more representative samples are necessary in order to confirm and possibly fine-tune the above evidence.
Another aspect that must be taken into consideration is the value of “green” properties and how real estate markets value them. These two elements may have a potential impact the collateral value (represented by the property) and therefore on the recovery rate realized from the property sale. The underlying assumption is that energy efficient properties have greater value than non-energy efficient ones (all the other features being the same) and that this value is less affected by the economic cycle (property resilience).
Contrarily to the probability of default, gaining robust evidence on recovery rates may prove rather difficult considering the average duration of the recovery processes in some European countries (e.g. Italy). Most financial institutions do not have EPC information at all, some others have started to collect it although only recently and then lacking it for most of the cases with complete workout processes for which recovery evidence is more robust.
The WP5 team is also facing this potential issue; completion of the data pool creation will tell how relevant this may be. Automatic Valuations Models (AVM) come into the game to help cope with such data limitations. The Automatic Valuation Model used by Crif, compliant with the the definition adopted by the European AVM Alliance®, is a system that provides an estimate of value of a specified property at a specified date (also in the past), using mathematical modelling techniques in an automated manner. The model does not necessarily require any previous values of the property to be provided as input, but it only requires that a property be specified and therefore they can function merely based on property address (or cadastral reference or other forms of unique property identification) and a few basic property characteristics. By analyzing these property attributes, (using the market comparison approach), it provides property valuation in different points in time allowing to observe how such value varies throughout time (possibly considering also impact of a full economic cycle).
A study has been carried out using a sample of 40.000 mortgage loans for which the ECP information was available. The property value corresponding to such loans was estimated for the years 2011-2018, separately for energy efficient and non-energy efficient properties. The preliminary evidence shows that energy efficient properties actually have different value trends and prove to be more resilient compared to the non-energy efficient ones. The expected “energy efficiency” effect on property value is illustrated in the exhibit.
We are at the very early steps of WP5’s correlation analysis study. However, some preliminary analyses have provided some promising hints into how energy efficiency can affect credit risk and the ability to recover from property sale. The above studies were conducted on limited data samples and the gained evidence may be affected by the used data. The ground for the WP5 study has been prepared. The data gathering phase, the most crucial one, has already started with the aim of building a pool large enough to be representative of the market and to allow for robust analyses. Data availability and quality are crucial. WP5 team has then prepared a plan for data remediation and enrichment so as to effectively cope with potential data issues.
Further evidence will be made available as the study progresses.
Copyright © Energy Efficient Mortgages Initiative
The project DeliverEEM has received funding from the European Union’s LIFE 2023 programme under grant agreement No.101167431. The EeMAP, EeDaPP, EeMMIP projects have received funding from the European Union’s Horizon 2020 research and innovation programme under grant agreements No. 746205, No. 784979 and No. 894117 respectively
Cookie | Duration | Description |
---|---|---|
cookielawinfo-checkbox-analytics | 11 months | This cookie is set by GDPR Cookie Consent plugin. The cookie is used to store the user consent for the cookies in the category "Analytics". |
cookielawinfo-checkbox-functional | 11 months | The cookie is set by GDPR cookie consent to record the user consent for the cookies in the category "Functional". |
cookielawinfo-checkbox-necessary | 11 months | This cookie is set by GDPR Cookie Consent plugin. The cookies is used to store the user consent for the cookies in the category "Necessary". |
cookielawinfo-checkbox-others | 11 months | This cookie is set by GDPR Cookie Consent plugin. The cookie is used to store the user consent for the cookies in the category "Other. |
cookielawinfo-checkbox-performance | 11 months | This cookie is set by GDPR Cookie Consent plugin. The cookie is used to store the user consent for the cookies in the category "Performance". |
viewed_cookie_policy | 11 months | The cookie is set by the GDPR Cookie Consent plugin and is used to store whether or not user has consented to the use of cookies. It does not store any personal data. |